Felix Jentzsch, M.Sc.
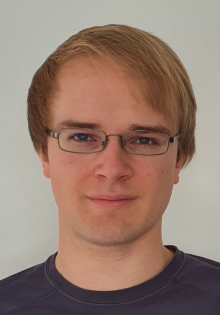
Member - Research Associate
33098 Paderborn
Book Chapters
A. Boschmann, L. Clausing, F. Jentzsch, H. Ghasemzadeh Mohammadi, M. Platzner, in: C.-J. Haake, F. Meyer auf der Heide, M. Platzner, H. Wachsmuth, H. Wehrheim (Eds.), On-The-Fly Computing -- Individualized IT-Services in Dynamic Markets, Heinz Nixdorf Institut, Universität Paderborn, Paderborn, 2023, pp. 225–236.
Conferences
F. Jentzsch, in: 2023 33rd International Conference on Field-Programmable Logic and Applications (FPL), IEEE, 2023.
H. Ghasemzadeh Mohammadi, F. Jentzsch, M. Kuschel, R. Arshad, S. Rautmare, S. Manjunatha, M. Platzner, A. Boschmann, D. Schollbach, in: Machine Learning and Principles and Practice of Knowledge Discovery in Databases, Springer, 2021.
Journal Articles
F. Jentzsch, Y. Umuroglu, A. Pappalardo, M. Blott, M. Platzner, IEEE Micro 42 (2022) 125–133.
Show all publications